erek
[H]F Junkie
- Joined
- Dec 19, 2005
- Messages
- 10,906
Interesting
"Hopefully, this was a quick and fun look at the NVIDIA DGX GB200 NVL72. For some who were unsure of what they were seeing, we had our original NVIDIA GTC 2024 Keynote Coverage that goes into many of the components. Still, it is cool to see in person and get a bit of a closer look."
Source: https://www.servethehome.com/this-is-the-nvidia-dgx-gb200-nvl72/
"Hopefully, this was a quick and fun look at the NVIDIA DGX GB200 NVL72. For some who were unsure of what they were seeing, we had our original NVIDIA GTC 2024 Keynote Coverage that goes into many of the components. Still, it is cool to see in person and get a bit of a closer look."
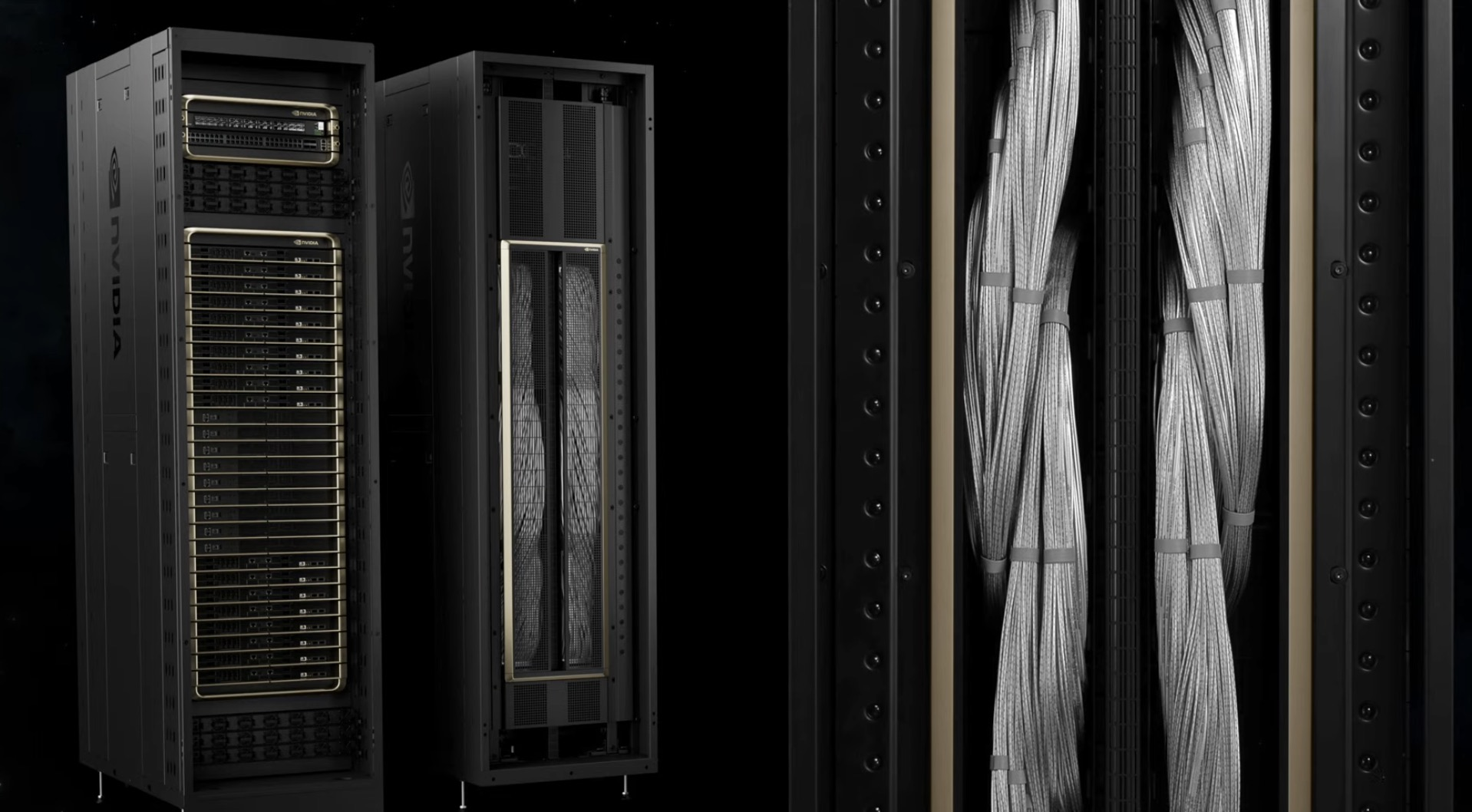
Source: https://www.servethehome.com/this-is-the-nvidia-dgx-gb200-nvl72/